What Can Industrial Software Vendors Learn From The Air Canada Chatbot Hallucination Case?
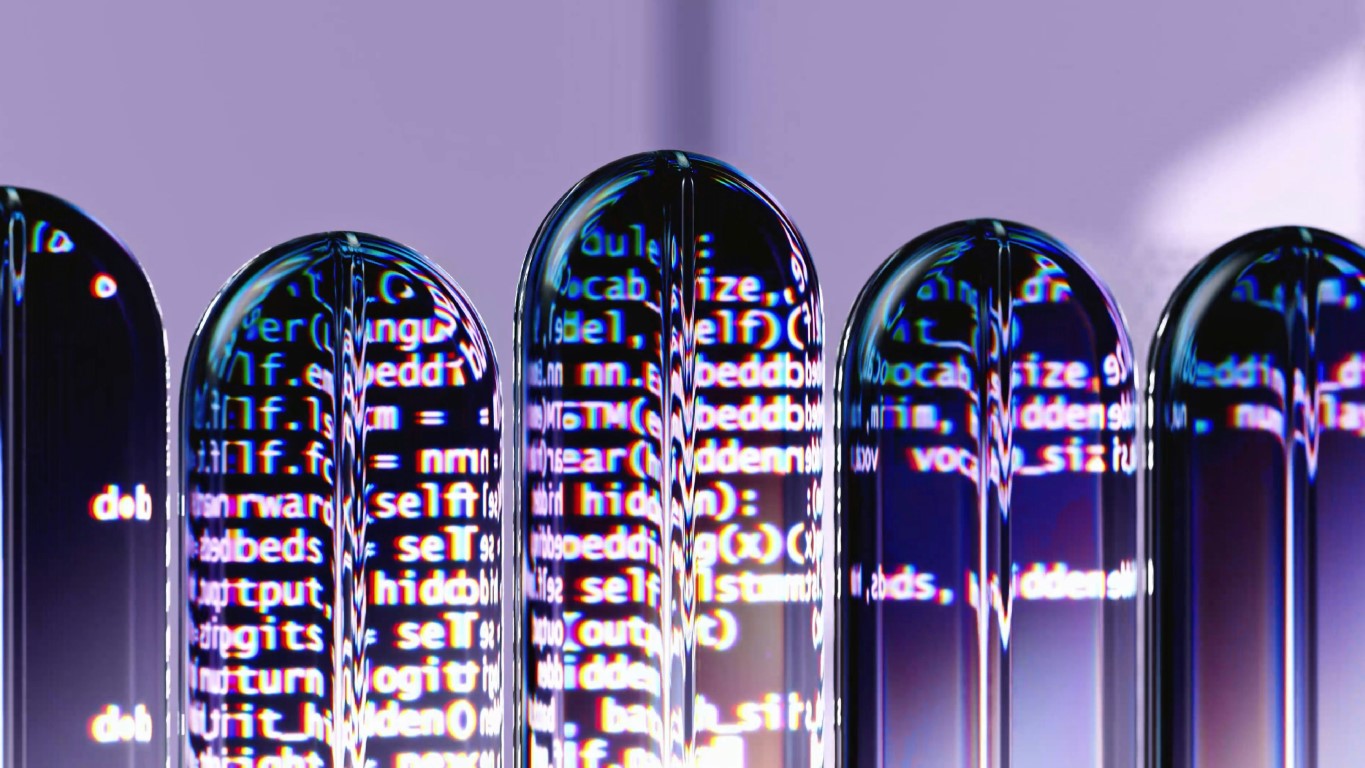
What Can Industrial Software Vendors Learn From The Air Canada Chatbot Hallucination Case?
In early 2024, the Air Canada fiasco served as a cautionary tale about the pitfalls of unregulated AI use. The airline faced repercussions when its AI chatbot provided inaccurate bereavement fare information, which led to a tribunal's decision mandating compensation for the misled passenger. This incident underscored the reputational risks associated with AI missteps and highlighted the urgent necessity for strict, ethical AI governance frameworks to avert similar issues in the future.
The growing integration of generative AI (GenAI) into industrial software firms’ product roadmaps is primarily motivated by the prospective improvements in worker engagement, decision-making and productivity. This integration is keenly focused on enhancing recommendation systems and deploying retrieval-augmented generation (RAG) for advanced information retrieval. Firms such as IFS and SymphonyAI are pioneering this movement, embedding GenAI copilots in solutions ranging from plant performance to ERP systems. The criticality of maintaining accuracy and transparency in AI-driven systems is evident, as trust in the AI’s output is fundamental to operational success. The consequences of inaccuracies or ambiguity in AI implementations could be extremely severe in industrial settings, emphasizing the need for comprehensive oversight to prevent substantial operational and safety issues in vital business functions.
Adopting robust frameworks such as explainable AI (xAI), knowledge graphs, and post-generation attribution and rationale models is essential as firms expand their use of GenAI. Explainable AI is indispensable for clarifying AI’s decision-making processes, rendering the complex algorithms understandable and accountable, which is key to building confidence and correcting any system biases or errors. Integrating knowledge graphs, as Cognite has done, enables GenAI copilots to effectively navigate complex data sets and improve information contextualization, thus minimizing hallucinations. Furthermore, implementing post-generation attribution and rationale, a strategy used by C3 AI for RAG systems, involves re-evaluating model responses for accuracy and editing unsupported content, enhancing decision-making quality and bolstering AI’s contribution to organizational productivity and informed decision-making.
In this transformative era, software vendors integrating GenAI must emphasize stringent safety, accuracy and transparency measures. This commitment is vital for responsibly harnessing AI's potential, ensuring that technological advancements are made with ethical integrity and establishing a standard for responsible AI innovation. It is a crucial time for firms to exemplify diligent AI deployment, thereby ensuring a future where technological progress aligns with the highest standards of reliability and ethical responsibility.
For more information on GenAI, read the following Verdantix reports: Market Insight: Understanding The Rapidly Evolving Landscape Of Generative AI; Market Insight: Ten Applications Of Large Language Models For Industry; Buyer's Guide: Industrial Data Management Solutions (2024).