Why DeepSeek’s R1 Is Actually Good News For Enterprises Everywhere
On January 27th, 2025, graphics process unit (GPU) behemoth NVIDIA saw nearly $600 billion wiped off its previous $3.6 trillion market capitalization. This is the biggest one-day drop in modern history – beating the previous record on 3rd September 2024, shortly after NVIDIA announced the lowering of its revenue growth expectations.
The trigger? An upload to GitHub and Hugging Face by Hangzhou- and Hong Kong-based quantitative hedge fund High Flyer Capital Management – or more specifically, its AI-focused research subsidiary DeepSeek. Headlines across the business world – as well as blogs, vlogs and even mainstream news – have been feverishly opining on DeepSeek’s new family of open-source ‘thinking’ large language models (LLMs).
Open-weight models such as those from DeepSeek, alongside Alibaba’s Qwen, Meta’s Llama, Mistral’s Mixtral and Microsoft’s Phi have long been darlings of the AI community. These offer developers the ability to download, fine-tune and deploy useful artificial intelligences securely on their own infrastructure. However, closed, API-only models from labs like OpenAI – from its 2019 second generative pre-trained transformer (GPT) to today’s GPT-4o and o1 – have still dominated the enterprise-grade echelon, alongside Anthropic and Google offerings.
Open-source models were rarely at the frontier level. The closest we saw was Llama-3.1-405B in July 2024, which rivalled the benchmark performance of Claude 3.5 Sonnet. Closed models quickly regained the crown, with OpenAI previewing its first ‘thinking’ model in September 2024 and Anthropic leapfrogging with an improved Claude 3.5 Sonnet in October. December, however, saw OpenAI announce a breakthrough with o3, beating Francois Chollet’s ‘memorization-proof’ ARC-AGI benchmark.
DeepSeek’s journey to disrupting Silicon Valley supremacy began in earnest back in February 2024 with an improved reinforcement learning algorithm applied to a mathematics-focused LLM, DeepSeekMath. The algorithm delivered gains compared with conventional methods, although in May DeepSeek-V2 continued to focus on low-cost distributed inference amid a price war for LLM endpoints in China.
DeepSeek released its first serious frontier contender, DeepSeek-V3, on Boxing Day, to intense fanfare within the AI community. This was apparently trained on a cluster of just 2,048 restrictions-compliant H800 GPUs for 2.8 million hours – which would cost, at retail, around $6 million – just one tenth of the 31 million H100 hours used to train a comparable model from Meta. Its technical report detailed an “internal DeepSeek-R1 model”, originally a closed preview from November, to improve chain-of-thought reasoning for DeepSeek-V3, resulting in a truly frontier-class conventional LLM.
Applying GPRO directly to the shiny new DeepSeek-V3, the (new) DeepSeek-R1 – open-sourced on 20th January and detailed in another technical report – is what sent Western AI labs into ‘panic mode’. It solves hard problems with better reliability than the level of OpenAI’s o1, while transparently showing human-readable ‘thinking’. The moat disappeared. Enterprises willing to spend $50-$100/hr on a private cloud H100 inference cluster can now serve hundreds of concurrent requests to deliver high-value use cases at vast scale – all while keeping all data within their private tenant.
The 2025 Verdantix survey of 304 industrial decision-makers finds that 68% of organizations lack trust in AI-derived insights. DeepSeek-R1’s frontier performance in benchmarks – both public and private – will translate to more transparent outputs at lower costs than comparable reasoning models, supporting firms in overcoming the ‘trust’ barrier. Even with distilled models capable of running on a single commodity GPU, Jevon’s paradox suggests the Cambrian explosion of use cases for cheap, reliable, bite-sized intelligence will once again saturate supply of inference GPUs – and the grid.
DeepSeek has successfully done for natural language what DeepMind did with the game of Go in 2016. Except this time, the world has use cases ready and waiting.
NVIDIA shareholders need not panic yet. Silicon Valley? I’m not so sure.
Enterprises everywhere rejoice.
About The Author
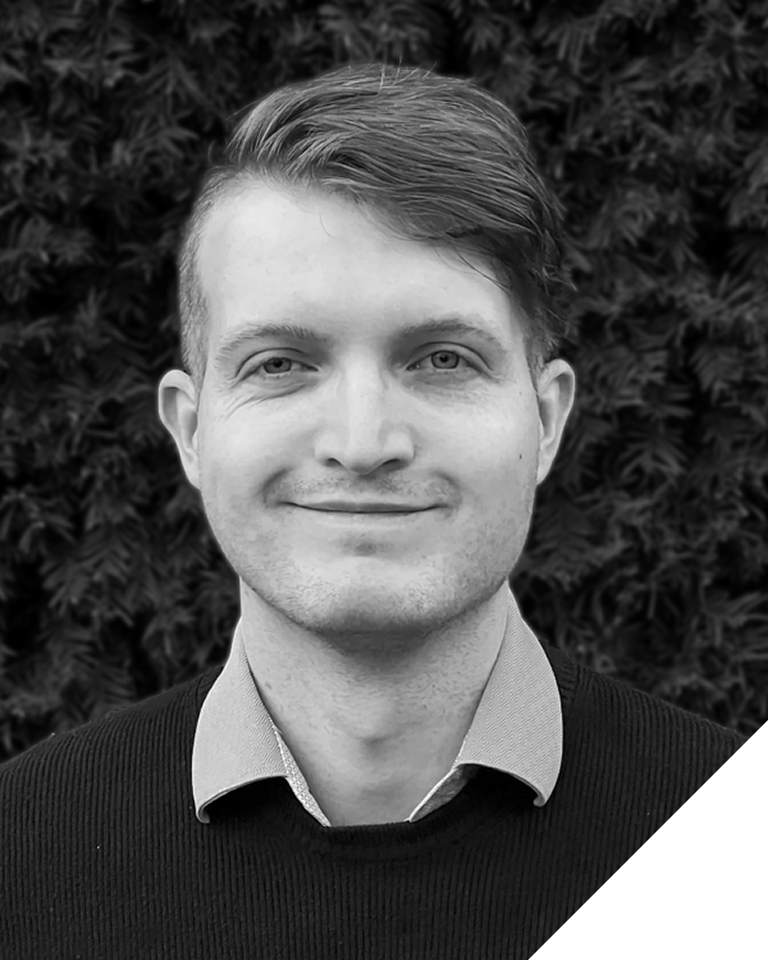
Joe Lamming
Senior Analyst