Senseye’s PdM Enterprise Open Platform Puts Customers In Control Of Their Predictive Maintenance Journey
The evolution of data science is reshaping the industrial maintenance world. The sharp rise of AI and decline of IoT device costs are enabling new value propositions and enhancements to complex asset reliability-centred tasks. On the back of that, the data-driven asset performance management (APM) solutions market is forecasted to grow at 12.1% CAGR between 2020 and 2025, despite this year’s spending flatlining.
More firms are crossing the chasm, shifting from traditional condition-based to predictive maintenance practices. Predictive maintenance can extend the assets’ useful life and increase uptime while reducing operational costs and risks. In the 2019 Verdantix global survey of 284 executives in operations, maintenance, and engineering roles, 93% stated that high costs from unplanned downtime is a ‘very important’ or ‘important’ factor in prioritizing spend on digital technologies for APM. Digitization of maintenance practices also means that there is now increasing involvement from customers’ IT and digital acceleration teams. These teams are keen to leverage the in-house knowledge of their assets, business processes and maintenance practices along with commercially available products to develop solutions that best fit their needs.
This demand for greater configurability as well as customization has triggered a shift towards platform-based software deployments, which can be readily integrated in the firm’s digital ecosystem and tailored to deliver bespoke maintenance solutions. Senseye, the UK-headquartered predictive maintenance software vendor, announced on November 19, 2020, the release of Senseye PdM Enterprise in response to this trend. The cloud-based platform has an open architecture and offers extensive integration as well as configuration capabilities, allowing the customer to decide how it is deployed, used, and extended. This way, Senseye ensures that its customers can intelligently exploit third party or in-house developed applications as well as existing IT infrastructure, which can now be bolted on the customizable platform and work in conjunction with Senseye’s core predictive maintenance solution in a consolidated manner.
Senseye has proven the value of AI-powered predictive maintenance and demonstrated how it can help industrial firms deliver on their asset maintenance goals. In October 2020, Senseye raised a seventh round of investment from NTT DOCOMO Ventures and Sony Innovation Fund, bringing the total investment raised to date to about $15 million. It has also forged strong partnerships with OEMs and digital solution providers, such as Bentley Systems, Schneider Electric, and Siemens. With the launch of its new product, Senseye has manifested a well-timed strategic position to exploit the burgeoning APM market opportunity by offering a flexible as well as scalable solution to accelerate its customers’ Industry 4.0 maintenance journeys.
To hear from industry experts, tech leaders, and Verdantix analysts about asset management strategies and initiatives, please register for the Verdantix virtual event Next & Best Practices: From Asset Reliability To Digital Twins.
About The Author
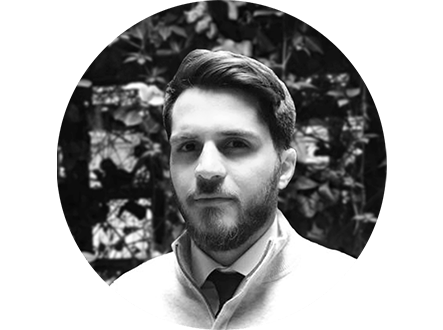
Victor Voulgaropoulos
Senior Analyst